Talk to an Expert
Machine Learning Fraud Detection and Prevention Guide
When shopping for a fraud prevention solution, you probably hear about machine learning-based fraud detection systems all the time. But does anyone really explain what machine learning for fraud detection is and why you need it? We know it can be a complex topic, so we’re here to break it down.
What is Machine Learning Fraud Detection?
Machine learning for fraud detection is the practice of using artificial intelligence to analyze data and learn from data to make informed decisions about fraudulent activity.
Some fraud prevention tools use supervised and unsupervised machine learning models. But most fraud providers only utilize supervised, which can weaken the tool’s ability to accurately decipher data and make informed decisions. The best fraud detection systems use both types.
What is Supervised Machine Learning?
Supervised machine learning is used to classify data or make predictions. When used for fraud detection, this type of machine learning looks at a user’s past actions — such as filing chargebacks, requesting refunds, or defaulting on loans — to make predictions about that user’s future behavior.
Supervised learning relies on well-labeled, extensive data to make accurate predictions. Essentially, that means fraud prevention providers need tons of historical data to create a successful system — especially providers that only use supervised learning.
What is Unsupervised Machine Learning?
Unsupervised machine learning uses algorithms (rule sets or processes) to analyze and group datasets. These algorithms then uncover patterns and trends without human intervention. That means it can evaluate a user’s current attributes — such as device type or number of email addresses associated with the user — to gauge potential risk.
Unsupervised learning is used less often in fraud detection software than supervised learning because of the complexity of its algorithms. However, the combination of both types of machine learning is what makes a fraud solution efficient, powerful, and accurate at detecting fraud and preventing it from happening.
The Difference Between Machine Learning and Artificial Intelligence
Machine learning is often used interchangeably with artificial intelligence (AI). However, they are not the same thing. Artificial intelligence refers to programming machines to think and act like humans. Machine learning is a subset of AI that acquires its own information and knowledge to make predictions.
Note: Machine learning also has its own subset called deep learning. Deep learning is what creates an artificial neural network — a layered structure of algorithms — that allows machines to make intelligent decisions on their own.
How Does Machine Learning Work?
Generally, the way that machine learning works is by collecting data, analyzing it, and using it to make predictions about user behavior. From there, the system makes decisions on whether to block or accept an interaction.
When we break that down further, it looks something like this:
- Users interact with your business. Interactions can include accessing an account, requesting a loan, or buying a product from an online store.
- Machine learning extracts data about users. The technology collects information about the user such as location, email address, type of device, and more.
- The data is analyzed by machine learning. Using one or both types of machine learning, the collected data is evaluated for patterns of behavior.
- Machine learning algorithms make predictions about users’ behavior. The technology identifies any risks associated with a specific user.
- Decisions are made. The technology approves or declines interactions based on policies and rules.
- Machine learning learns from each interaction. The technology records event outcomes and learns from them to improve decision accuracy consistently over time.
The Benefits of Machine Learning-Based Systems
Machines can process large amounts of data very quickly — which is why machine learning-based fraud systems are more effective, accurate, and consistent than manual fraud management practices. Plus, these systems are able to conduct extensive data analysis that just isn’t possible for humans to do.
Using machine learning in fraud detection relieves you of the hassle and resources needed to keep fraud in check — which means you:
- Gain back time and resources: Machine learning works faster and more efficiently than humans, so you can reduce labor-intensive, time-consuming manual processes and spend more time on revenue-generating tasks.
- Get better fraud detection: Machine learning can detect patterns and trends that humans simply can’t see. Plus, it learns from every interaction so outcomes are always improving — giving you the best possible fraud prevention.
- Are always protected: Algorithms don’t need breaks or sleep. They work 24/7, giving you never-ending protection.
- Receive accurate results: Because machine learning acts on facts — not subjective biases, assumptions, or opinions — it makes accurate decisions without the risk of human error. Plus, it analyzes data in real time, so decisions are based on current information.
Use Cases: Examples of Machine Learning for Fraud Detection
Want to know more about the benefits of machine learning-based fraud prevention? Kount has helped thousands of companies reduce manual processes and gain back time to focus on growth. Check out some of our client testimonials to see for yourself.
CASE STUDY
Automation helps Central Restaurant Products grow
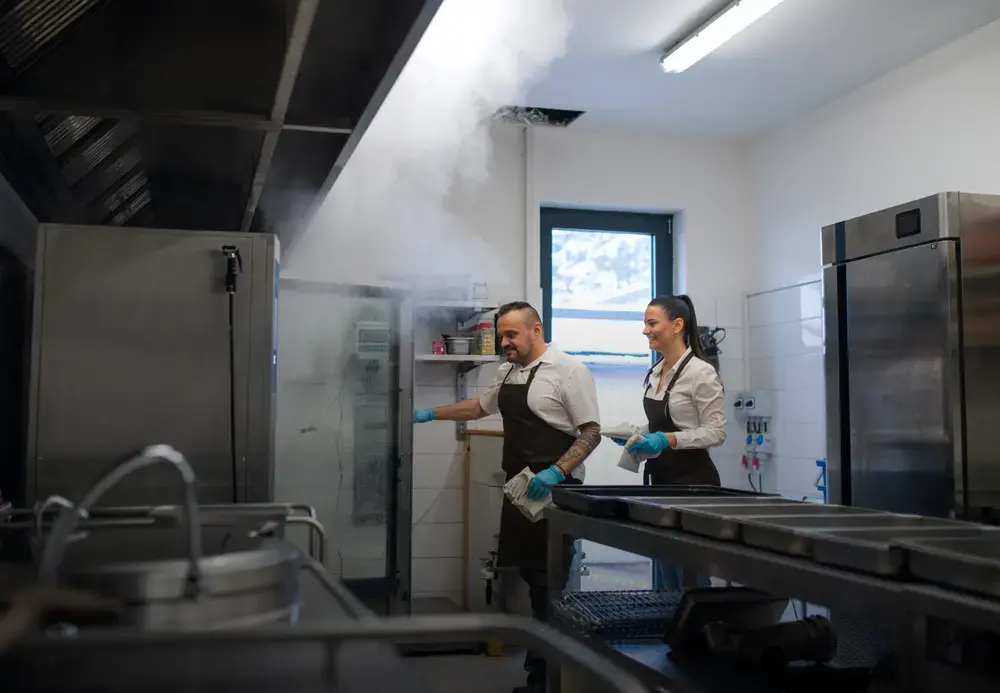
Before using Kount, Central Restaurant Products manually reviewed every order. But once the business started growing, it was difficult for them to keep up. With the aid of Kount’s adaptable machine learning, they were able to significantly reduce manual processes and accept more orders.
CASE STUDY
Fetch Rewards reduces friction with machine learning
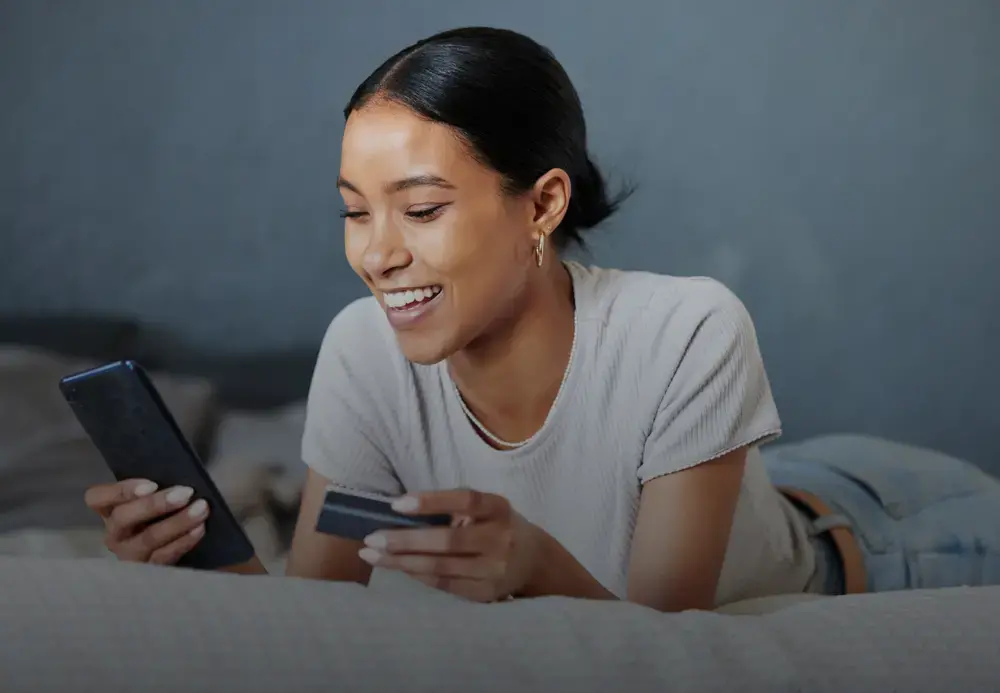
When Fetch Rewards came to Kount, they were manually reviewing hundreds of thousands of transactions — an inefficient process. Thanks to Kount, they were able to replace manual processes with automation. And as they gained more data, they were able to reduce friction for good customers while approving more transactions.
TESTIMONIAL VIDEO
GNC leverages machine learning to fight fraud
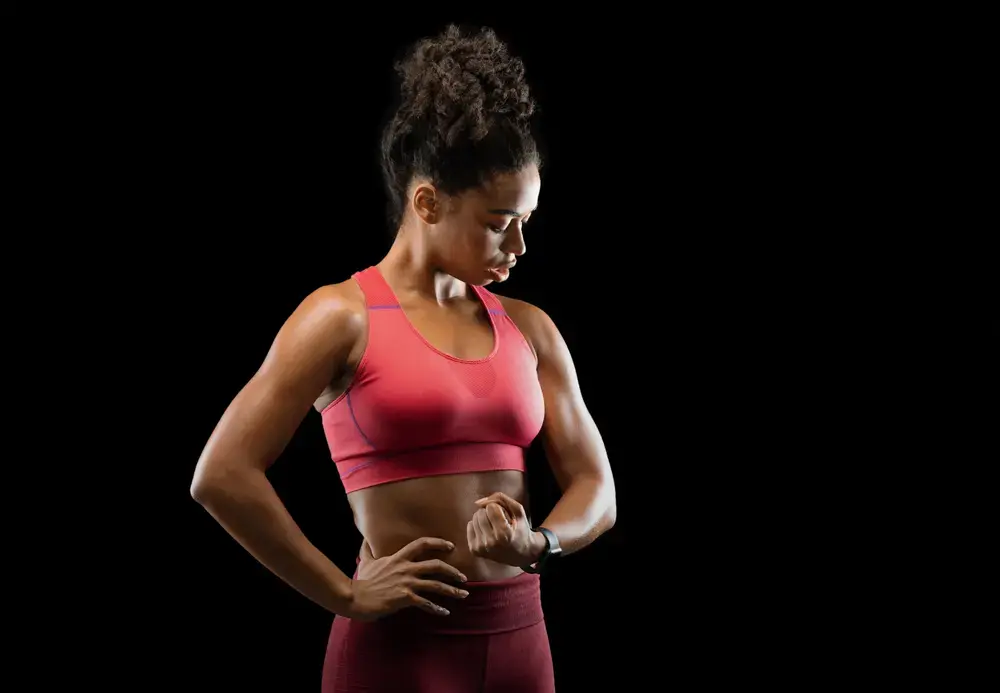
When it comes to fraud, GNC’s main goals are to protect the brand from emerging threats and keep customers safe when shopping online. And with Kount’s adaptable AI and machine learning, the company is able to accomplish that goal.
What to Look for When Buying Machine Learning for Fraud Detection
There are multiple fraud providers on the market. Picking the right one can be a lengthy and challenging process — unless you know exactly what you are looking for.
Uses multiple types of machine learning
Many providers only use one type of machine learning. But to get the best protection possible from a fraud tool, look for a provider that uses both supervised and unsupervised learning.
Think of it this way: gas cars are great, but can be expensive to fuel up. However, hybrid cars are typically more fuel-efficient — saving you money while still giving you the experience of a gas car.
Lets you customize policies
Your business has unique challenges. So you need a solution that can adapt to your business, not the other way around. Make sure you work with a provider that lets you determine the rules and policies that fit into your fraud strategy.
Gives you flexibility
What works for other businesses might not work for yours. Maybe you don’t have the time or resources to manage fraud at all. Or maybe you still want to review certain orders and let automation tackle the rest. Find a provider that can accommodate as much or as little automation as you need.
Collects robust data
Machine learning can only be as good as the data that fuels it. When looking for a provider, ask about the data they collect. Do they have decades-worth of data and experience or are they a newer provider? Do they collect information about users, devices, transactions, payments, and locations or do they only collect transaction details or payment data?
Offers guidance from fraud experts
No matter how “simple” and “easy” a fraud detection system may be to use, there might be times where you need a little bit of guidance. That’s why you want to work with a provider that provides additional services beyond fraud protection. A truly beneficial solution should come with fraud expertise from real humans.
FAQs About Using Machine Learning for Fraud Detection
Need to know more? We’ve answered the most common question we get about machine learning for fraud detection.
Does machine learning cost more?
The simple answer is no — it doesn't cost more.
But to break it down, take this scenario:
Say it takes an employee one minute to complete a manual review. Hypothetically, that employee could complete 60 reviews in one hour. Let's say the employee is paid $25 per hour. That means each review costs about $0.42.
But humans need breaks. And sometimes they make mistakes.
On the other hand, technology might cost $0.07 for each transaction review. In one hour alone, you've saved $20. An employee would have to review 857 transactions each hour to be as cost-effective as machine learning.
Plus, technology doesn’t need breaks — it can work around the clock.
So even the most expensive technology is still more cost-effective because it provides better, more accurate protection in significantly less time than error-prone, labor-intensive processes.
Take Kount, for example. On average, Kount saves businesses 40% on labor costs and 84% on labor hours — all thanks to machine learning.
Will I have a higher rate of false positives?
No. Because machine learning can pick up on trends and patterns that humans can’t catch with manual reviews, you should have more accurate results — thus, less false positives. In fact, on average Kount helps businesses reduce false positives by 70% while still blocking the greatest amount of fraudulent transactions.
Does machine learning slow down the decision making process?
No. Machine learning does the exact opposite — it speeds up the decision making process with better accuracy. It analyzes data within milliseconds — less time than it takes to blink an eye — and delivers immediate results so customers don’t experience any delays no matter what stage they’re at in the customer journey.
Kount has an average uptime of 99.99%. That means the technology is essentially always working.
What happens to manual reviews?
If you like to have complete control over your business, don’t fear. Machine learning can be customized to fit your needs. If you still want to manually review certain orders, all you have to do is create policies that will set aside orders that meet specific criteria.
On the flipside, if you want to completely eliminate manual reviews, machine learning can help you do that too. It all depends on the policies and risk thresholds you set up.
What is the difference between a rules-based system and a ML-based system?
Rule-based fraud detection relies on sets of rules to detect and make decisions about fraud. Common rules include location, frequency, and user history. The technology blocks or accepts transactions depending on whether or not a transaction fulfills the rules. This system requires frequent intervention from humans to quality check and update rules as the system collects more data.
Machine learning-based fraud detection operates similarly; however, it requires no intervention from humans. Because machines constantly learn from interactions, they can improve processes on their own. As a result, ML-based systems significantly cut down the cost of fraud management — saving money in overhead and labor.
Plus, with a ML-based system, you can write your own rules that fit your unique business situations. Most rule-based systems have pre-packaged rule sets that leave little room for customization.
Ready to get started?
Kount is the industry’s leading trust and safety technology solution. We’ve been using machine learning for decades — ensuring you get the best results possible. Sign up for a demo today to learn more.